E'kabel Blog
O&G industry can save 90 million euros per year
Oportunidades de Aplicación de Algoritmos Predictivos en la Optimización de Producción de Campos Petroleros
En el primer semestre del 2020 la Industria del Petróleo y el Gas Natural se vio afectada por la contracción de la economía generada por la pandemia a nivel mundial, el precio del barril de petróleo llegó a valores por debajo de los 30 dólares e incluso estuvo en cifras negativas [1], este fenómeno sin precedentes impulsó la búsqueda de nuevas estrategias e inclusión de tecnologías.
Empresas como Abu Dhabi National Oil Company, Total, Shell y la Dirección de Petróleo de Noruega han acelerado la migración hacia la transformación digital y el uso de la ciencia de datos, gracias al avance en las telecomunicaciones, los servicios en la nube y los desarrollos previos de la inteligencia artificial (IA).
En América Latina la integración de la IA también ha considerado aspectos sociales; en algunos países han utilizado este enfoque para mejorar la percepción de las comunidades hacia la industria petrolera, por medio del desarrollo de metodologías pedagógicas aplicadas en el relacionamiento comunitario, logrando incrementar en un 45% el nivel de aprobación de las comunidades hacia las operaciones de exploración y producción [16 -17].
Hablar de pozos y campos inteligentes, hace referencia a los activos que logran un nivel de autonomía y optimización, a través de la integración de conocimiento, tecnología y flujos de trabajo para gestionar y mejorar la eficiencia de las operaciones, logrando maximizar el ciclo de vida del campo.
La IA es aplicable tanto a la optimización como al mantenimiento y se ha incorporado en las metodologías de trabajo para apoyar en la búsqueda de soluciones y disminución de los eventos que ocasionen pérdida de producción; gracias al uso de la telemetría para la medición remota se ha facilitado el acceso a grandes cantidades de datos que pueden ser
aprovechados para el entrenamiento de los algoritmos predictivos; estudios indican que al realizar la proyección económica de aplicar la IA en identificación y prevención de fallas, se estima que la tecnología evita pérdidas cercanas a 90 millones de euros al año[2] gracias al impacto de la priorización y planificación de mantenimiento en sistemas de levantamiento artificial, que de una forma tradicional se reflejarían en paradas no planificadas y acciones reactivas ante las fallas.
Para la implementación de estas metodologías es necesario que la adquisición de datos se realice en tiempo real, la misma debe asegurar: resistencia, confiabilidad y durabilidad ante condiciones operacionales extremas, pozos altamente desviados, completaciones multilaterales o aplicaciones no convencionales; el valor de tener información en tiempo real es equivalente a una reducción de costos del 30% anual en comparación con el uso de métodos tradicionales como el registro de producción PLT (Producction Logging Tools)[3], debido a que disminuyen el tiempo de toma de decisiones y mejoran la capacidad de gerencia del yacimiento.
«Research shows that AI can help the industry achieve savings of 90 million euros per year…»


Una de las aplicaciones de la IA más utilizada en el sector Oil and Gas es el aprendizaje automático, también conocido como Machine Learning (ML), ya que tiene la capacidad de acceder a los datos históricos y aprender de las diferentes experiencias operativas, para generar lo que conocemos como: Algoritmos Predictivos.
Algoritmos Predictivos
Estos buscan predecir el funcionamiento de los equipos y emitir alertas tempranas; para lograr estos resultados el algoritmo debe pasar por un proceso en el cual se integran
los siguientes aspectos:
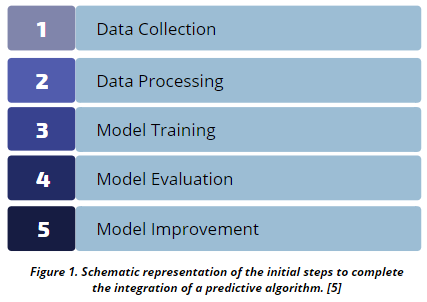
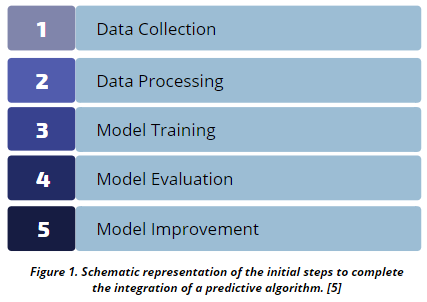
El desarrollo de un algoritmo efectivo, parte de la recopilación y suministro de información histórica (producción, fallas, condiciones operacionales, datos de
sensores, etc.), luego estos datos se procesan, asegurando su calidad, y se prosigue con el entrenamiento del algoritmo, para ello se realizan diversos escenarios de prueba con la finalidad de evaluar y mejorar el modelo, garantizando predicciones con el menor grado de incertidumbre posible, también se mide la efectividad del reconocimiento de patrones y la asertividad de alertas generadas.
La evaluación del modelo implica probar el algoritmo en un grupo de pozos o porción de los datos apartado para este fin, de esta manera él puede comparar las predicciones
realizadas con los datos que recibe en tiempo real esto permite evaluar la efectividad del modelo para identificar problemas; terminado este periodo se pueden realizar las modificaciones que sean necesarias para finalmente implementar el algoritmo como una parte integral de las operaciones.
Es importante mencionar que antes de poder considerar autónoma una operación o proceso en pozos y/o campos petroleros deben existir ciertos niveles de desarrollo, la tabla 1 muestra una de las clasificaciones más comunes:


Siendo el suministro de datos la base que permite a los algoritmos recopilar y usar la información; es necesario que las instalaciones petroleras cuenten con los niveles 1 y 2 de desarrollo, los equipos de adquisición en fondo, el Sistema SCADA (Supervisory Control And Data Acquisition) y la nube, son algunos ejemplos de los factores que hacen viable el acceso a la información y su envío para el uso en forma remota, generando que la información esté disponible en cualquier dispositivo conectado a la red corporativa; de esta manera el algoritmo puede tener acceso a las variables que le permiten hacer el reconocimiento de patrones y predicciones lo más confiables posible.
Los beneficios principales de implementar esta tecnología son:
- Disminución de costos asociados a pérdidas de producción por paradas no programadas.
- Optimización de parámetros operacionales y planeación eficiente de mantenimientos preventivos.
- Obtención y priorización de alertas en función de los eventos que necesitan atención urgente.
- Apoyo al especialista en la toma de decisiones, permitiendo acciones más rápidas y eficientes.
- Prevención de daños a causa de problemas derivados de malas prácticas o fallas inminentes.
- Reconocimiento de patrones operacionales, que generalmente no son evidentes.
Identification and Prognosis of Failures in Artificial Lift Systems
About 90% of the wells in a field go from producing by natural flow to producing by some artificial lift system. In those cases, conventional optimization practices contemplate the acquisition of downhole data, allowing the operator to improve, fine-tune and get to know the operational behavior of the artificial lift system.
This data feeds predictive algorithms, enabling early detection of failures, prioritization of anomalies, and rapid decision-making.
This practice focuses on reducing unplanned deferred production due to unforeseen events. An example is a variation in the operational parameters of electro-submersible pumping systems (BES) connected to variable frequency drives (VFD) on the surface. In these cases, the information is centralized in SCADA and sent to the control room.
Among the main variables that the algorithm would use to detect a fault are:
- Annulus pressure
- Pressure on the tubing
- Revolutions per minute (measured in the VFD)
- Pressure and temperature of the oil in the pump
- Motor temperature
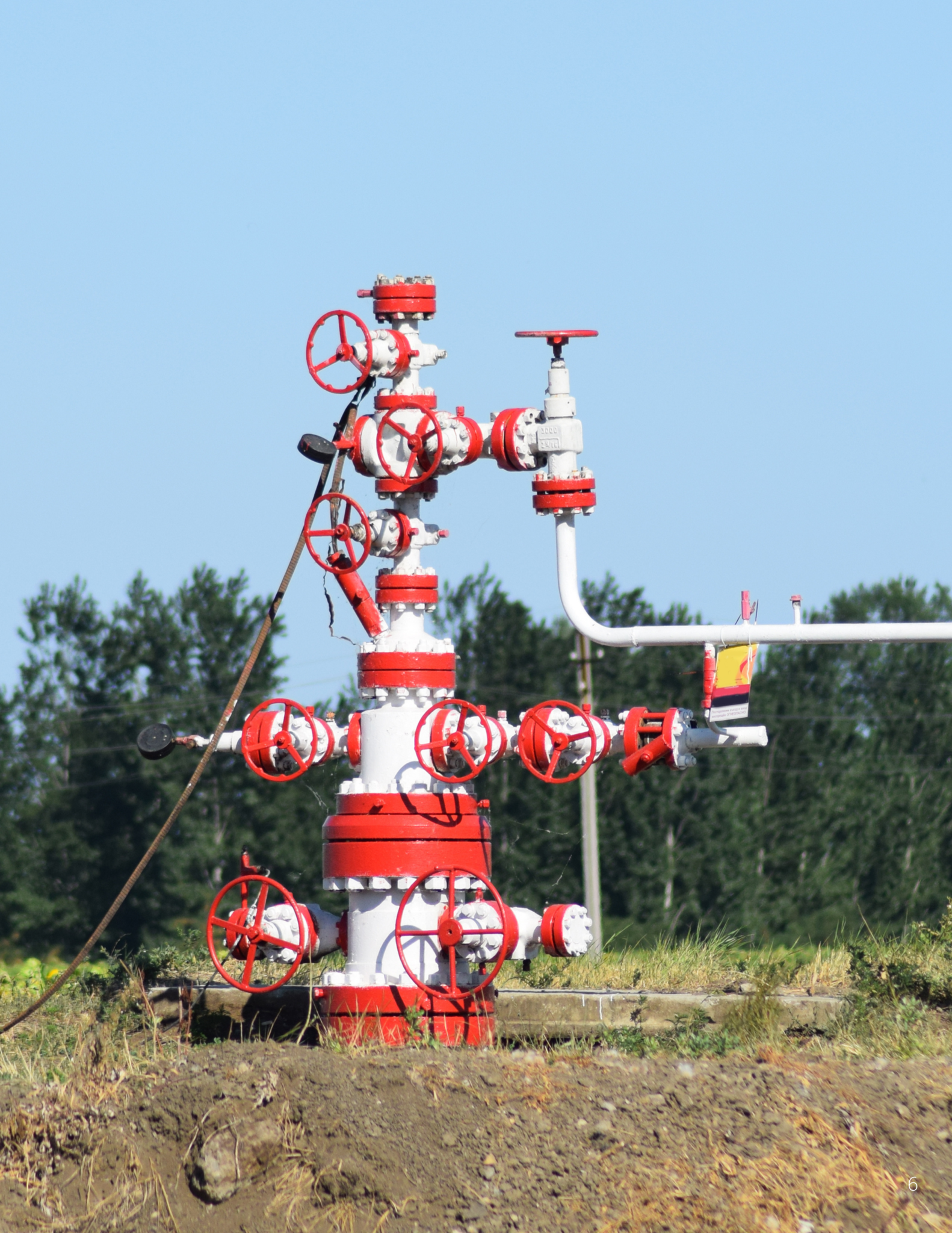
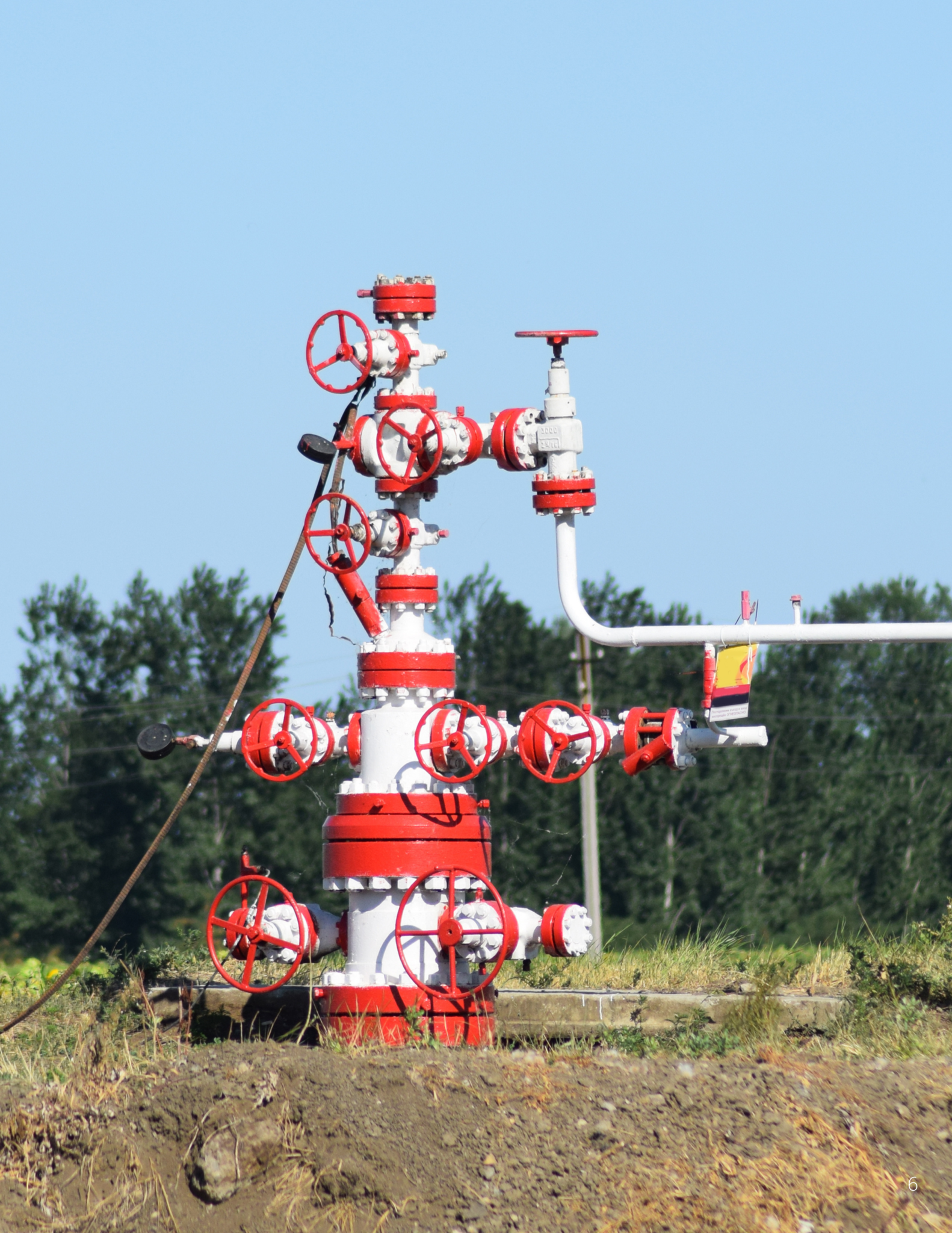
Depending on the configuration, this information is transmitted in seconds or minutes. Once the algorithm receives it, it compares it with the baseline or optimal operation state. If it detects any variation that cannot correct remotely, it will raise the alarm allowing engineers to take action.
Another example is the unscheduled shutdowns of gas compression plants, which interrupt the operations of gas-lift wells. With the help of ML, companies can prevent economic losses and support future intervention planning. Engineers can install ML technology from the plant to the wells, and then it is possible to maintain an optimal injection rate and extend the useful life of the equipment. Studies show that AI applied in wells with artificial lift systems has managed to forecast events between 12 and 60 days before they occur [2-8], prioritizing the failure with the highest probability of occurrence according to the recognized pattern and indicating the preventive maintenance or the solution required for each alert.
Challenges of applying AI in the Selection of Recovery Methods
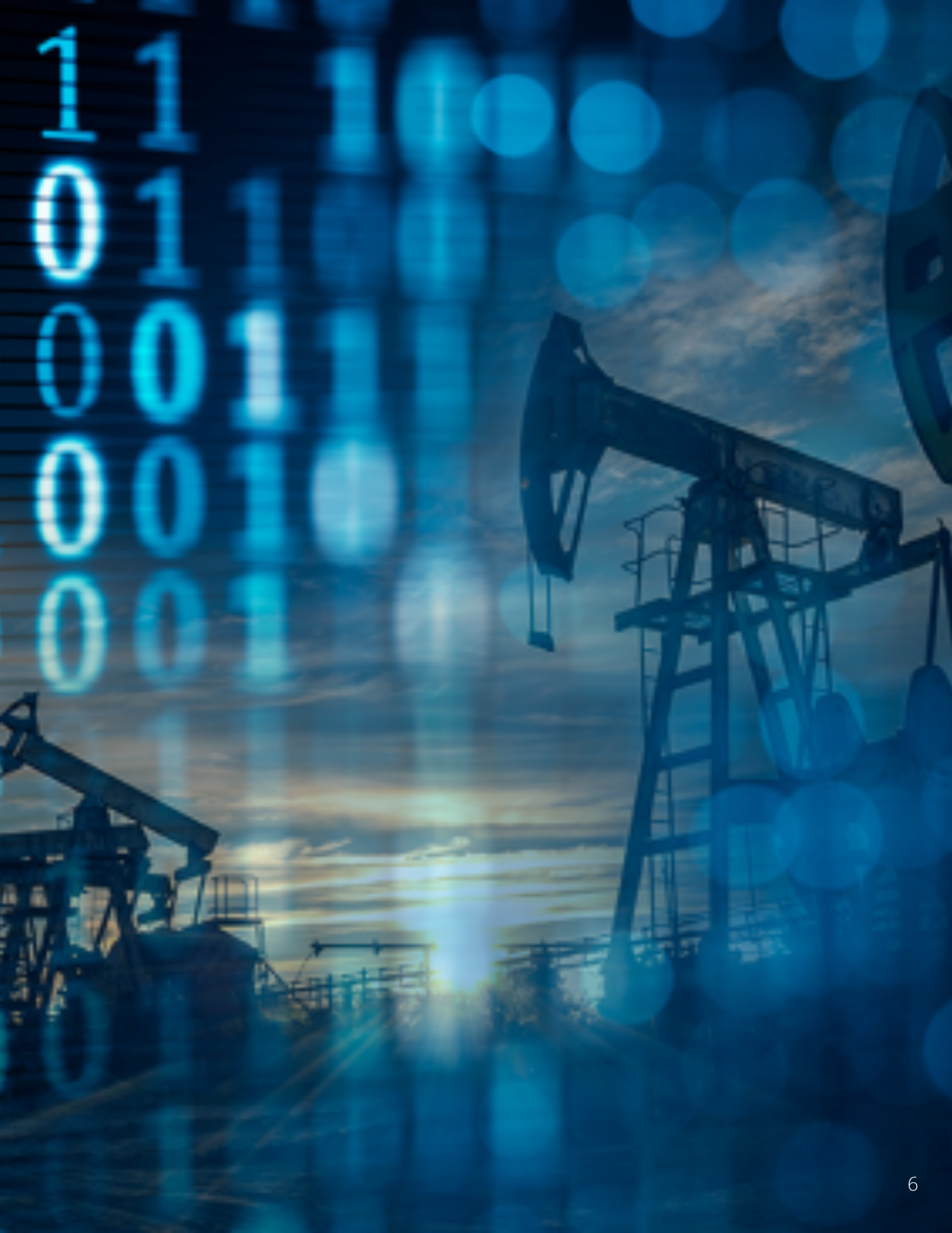
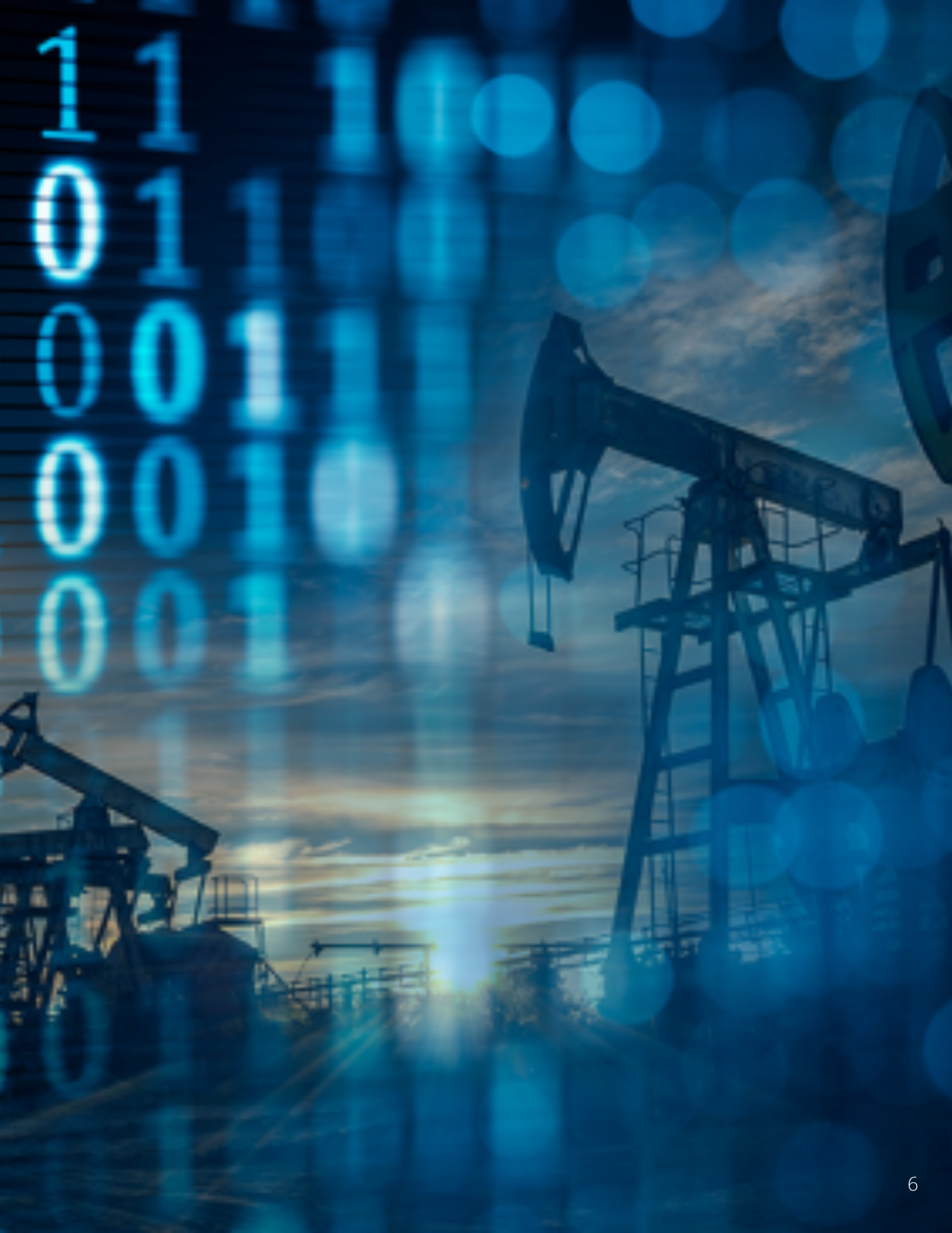
Generally, a field produces around 10% of its total reserves through primary recovery. Then to extend its useful life, secondary or tertiary recovery methods are usually implemented to obtain between 30 and 60% of recoverable reserves.
In these cases, the implementation of AI supports decision-making related to selecting the most appropriate method.
To achieve it, AI uses some of the following tools:
- Well interconnection maps
- Historical production data
- Pressure test information
- Reservoir characteristics
- Probabilistic relationships between the main variables of the field and the applicable recovery methods.
Then, AI can use other predictive algorithms to verify operational variables related to pressure and injection rate, steam quality (thermal cases), and energy consumption. That data helps optimize processes to maintain production at an economically viable standard.
The algorithm requires not only historical data but also background and surface information during the operation, in which case the main challenges are related to data acquisition.
In highly deviated wells, where it is necessary to consider the resistance of the materials, a widely used alternative is the integration of fiber optics as a sensor medium and an interrogator to monitor and issue alarms if parameters outside the operational range are identified.
This technology uses distributed detection, which allows obtaining a temperature profile and even seismic data along the entire length of the cable. In the case of steam injection wells, it will enable the use of Distributed Temperature Detection (DTS) to identify if there is an alteration in the quality of the injected steam, to know the temperature and the behavior of the steam front through the monitoring of observer wells[10].
Fiber optics can also monitor the behavior of mandrels in gas injection wells and use the Joule Thompson effect of gas cooling to determine the system’s efficiency qualitatively. Suppose there is a gas slug or change at the mandrel level [9]. In that case, it is possible to identify a time-dependent thermal pattern associated with the fault, which becomes recognizable to the algorithm. That information would save time through the multi-well analysis.
The optimization of the recovery method requires well-defined parameters; however, each field and well will have a particular behavior. For this reason, personalized models that select recovery methods based on each field’s data represent an improvement of significant impact on the industry. Algorithms can be trained and corrected in programming languages such as Python and then used once the accuracy valuesare acceptable, reducing uncertainty about a decision that requires investment for the operating companies.
Recommendations
After evaluating the benefits and the application range of AI, the first step, if someone seeks to implement best practices in a production process, is to define both the scope and the resources that will be part of the project (specialists, wells, fields, etc.).
Then it is crucial to integrate a team that knows how to apply predictive algorithms and AI in the oil industry. The team must prepare a pilot test, and after a reasonable testing time, it will be possible to extend the use of AI to the rest of the areas that require this support.
Conclusions
AI deployment and accelerated digital transformation can improve the competitiveness of the oil & gas sector in the global energy industry.
Machine Learning can potentially address the challenge of turning historical information acquired during operations into barrels, positively impacting production and field management.
Using these technologies makes it possible to integrate the cloud, machine learning predictive algorithms, and data management experience to implement best practices in energy.
References
[1] Retrieved from: [https://datosmacro.expansion.com/materias-primas/brent]. March 2021.
[2] Retrieved from: [https://bakerhughesc3.ai/customers/downtime-high-value-offshore-wells/ ] March 2021.
[3] Continuous Intelligence in charging Reservoirenvironments. Real-time reservoir and production data via capillary-conveyed technology. Weatherford 2020.
[4] REDUTSKIY, Y. Conceptualization of Smartsolutions in oil and gas industry. The 7th International Conference on Sustainable Energy Information Technology (SEIT 2017). Molde University College. Norway.
[5] ARIAS, J. Estado del Arte y Marco conceptual de las tecnologías de Machine Learning aplicadas al recobro mejorado. Grupo de Investigación GICBA de la Unidad Tecnológica de Santander. May 2019. Colombia.
[6] FAHAD, I. MUHAMMED A., AMIRMASOUNDK., SHAHIN, N. Artificial Lift optimization using machine learning applications. August 2020.
[7] JANSEN VAN RENSBURG, N. Autonomous Well Surveillance for ESP Pumps Using Artificial Intelligence. Siemens AG Germany. 2019.
[8] JANSEN VAN RENSBURG, N. KAMIN, L., DAVIS R. Using Machine Learning Based Predictive Models to enable preventative maintenance and prevent ESP Downtime. Siemens AG. Germany 2019.
[9] ELIZARRARAS A., MURILLO G., LOZADA M., VEGA A., Aplicación de Fibra Óptica en perfiles de producción. CIPM. Mexico 2005.
[10] Retrieved from: [https://globalenergy.mx/noticias-especiales/columnas/la-inteligencia-artificial-apuesta-obligada-para-la-industria-del- petroleo/#:~:text=En%202019%20la%20inteligencia%20artificial,d%C3%B3lares%20para%20fines%20d e%202025.] March 2021.
[11] CAMPETROL. Balance Petrolero. Cierre de 2019 e Incertidumbre 2020, la nueva conyuntura de precios y los desafíos del COVID-19. Colombia 2020.
[12] CHINN M., WUTKEWICZ M. Artificial Intelligence drives early-warning alerts to prevent ESP disruptions. Digital Transformation. World Oil. January 2019.
[13] IBARGUENGOYTIA P., BALLESTEROS A., GARCIA U. La toma de decisions para intervenir Pozos mediante técnicas de inteligencia artificial. ResearchGate. March 2015.
[14] CARRION F., LLIGUIZACA D., PERERO D. Selección de Métodos de Recuperación Mejorada de Petróleo utilizando el algoritmo de Machine Learning de los K vecinos más cercanos (KNN). July 2010.
[15] Retrieved from: [https://market.biz/report/global-ai-in-oil-gas-market-gm/572604/#details] March 2021.
[16] GUEVARA I, PASTOR P., SAAVEDRA A. Changing the negative perception of the Oil and Gas Industry in Colombia, Through an Energy Education Methodology. SPE, Gran Tierra Energy. Octuber 2019.
[17] GUEVARA I, PASTOR P., SAAVEDRA A. Data Science applied to pedagogical methodologies focused on changing the negative perception of the Oil and Gas Industry in Colombia. Glouphi, SPE Occidental Petroleum Corporation. Octubre 2019. PE. Octuber 2019